
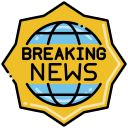
But going peacefully on the streets is only successful if the government you want to send a message to is listening, i.e. if it either cares for their citizens or is in any way rational.
I hope I’m wrong here, but I can’t see anything changing for the better in your country. It currently looks like 70 million people trying to talk through a knife fight.
I had this hope too, but he already went light-years too far and all I read is “soon …”.
I’m generally against violence, but I really hope you guys do something over there…